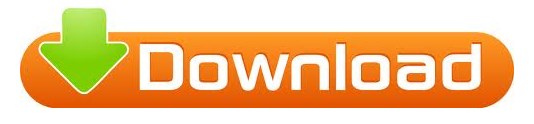
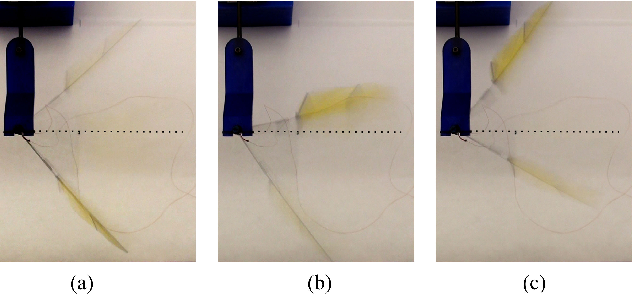
M., “ Two-Level Multifidelity Design Optimization Studies for Supersonic Jets,” Journal of Aircraft, Vol. 46, No. 3, 2009, pp. 776–790. and Wintzer M., “ Multifidelity Design Optimization of Low-Boom Supersonic Jets,” Journal of Aircraft, Vol. 45, No. 1, 2008, pp. 106–118.
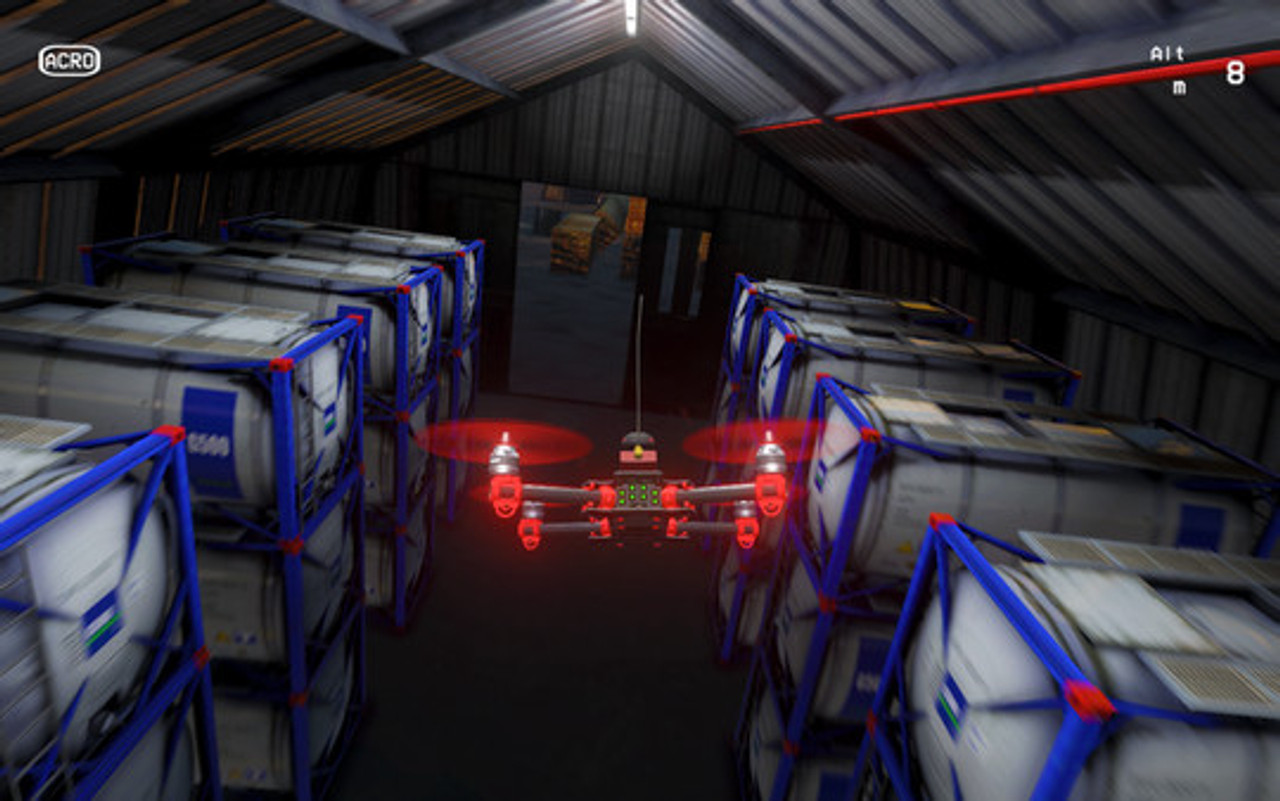
and Haftka R., “ Multifidelity Response Surface Model for HSCT Wing Bending Material Weight,” 7th AIAA/USAF/NASA/ISSMO Symposium on Multidisciplinary Analysis and Optimization, AIAA Paper 1998-4804, 1998. and Beran P., “ Bayesian Low-Fidelity Correction Approach to Multi-Fidelity Aerospace Design,” 58th AIAA/ASCE/AHS/ASC Structures, Structural Dynamics, and Materials Conference, AIAA Paper 2017-0133, 2017. I., “ Multi-Information Source Optimization,” Advances in Neural Information Processing Systems 30, edited by Guyon I. J., “ A Knowledge-Based Approach to Response Surface Modelling in Multifidelity Optimization,” Journal of Global Optimization, Vol. 26, No. 3, 2003, pp. 297–319. J., “ Wing Optimization Using Design of Experiment, Response Surface, and Data Fusion Methods,” Journal of Aircraft, Vol. 40, No. 4, 2003, pp. 741–750. and Collis S., “ Second-Order Corrections for Surrogate-Based Optimization with Model Hierarchies,” 10th AIAA/ISSMO Multidisciplinary Analysis and Optimization Conference, AIAA Paper 2004-4457, 2004. and Newman P., “ Approximation and Model Management in Aerodynamic Optimization with Variable-Fidelity Models,” Journal of Aircraft, Vol. 38, No. 6, 2001, pp. 1093–1101. and Torczon V., “ A Trust-Region Framework for Managing the Use of Approximation Models in Optimization,” Structural Optimization, Vol. 15, No. 1, 1998, pp. 16–23. and Gunzburger M., “ Survey of Multifidelity Methods in Uncertainty Propagation, Inference, and Optimization,” SIAM Review, Vol. 60, No. 3, 2018, pp. 550–591.
#Liftoff simulator wing series
and Keane A., “ Multi-Fidelity Optimization via Surrogate Modelling,” Proceedings of the Royal Society of London, Series A: Mathematical, Physical and Engineering Sciences, Vol. 463, No. 2088, 2007, pp. 3251–3269. N., “ Alternative Trim Analysis Formulations for Vehicles with Redundant Multi-Axis Control Surfaces,” Journal of Aircraft, Vol. 53, No. 1, 2016, pp. 60–72. and Taquin G., “ Multicontrol Surface Optimization for Blended Wing–Body Under Handling Quality Constraints,” Journal of Aircraft, Vol. 55, No. 2, 2018, pp. 638–651. and Princen N., “ Control Allocation Challenges and Requirements for the Blended Wing Body,” AIAA Guidance, Navigation, and Control Conference and Exhibit, AIAA Paper 2000-4539, 2000. H., “ Design of the Blended Wing Body Subsonic Transport,” Journal of Aircraft, Vol. 41, No. 1, 2004, pp. 10–25. The presence of even sparse high-fidelity data is key to reducing the variance in the overall analysis, thereby improving the quality of the predictions needed to inform early stage design decisions. The results show that low-fidelity models are enhanced by the presence of higher-fidelity data in key areas of the design space. In this paper, the approach is demonstrated on the stability and control analysis of a Blended-Wing-Body aircraft’s center-of-gravity limits, considering the longitudinal criteria of fly-to-stall, stall recovery, nose-wheel steering, and nose-wheel liftoff. The methodology is broadly applicable across engineering design problems where multiple information sources are available to support design decisions. The resulting multifidelity surrogate provides a rapid analysis capability, which in turn enables the high number of evaluations needed to conduct multidisciplinary trade studies and to propagate uncertainty to the overall system level. This weighting takes into account both the quality of the Gaussian process approximation and the designer’s confidence in the underlying information source being approximated. The multifidelity data fusion is achieved using a fidelity-weighted combination of Gaussian process surrogate models.
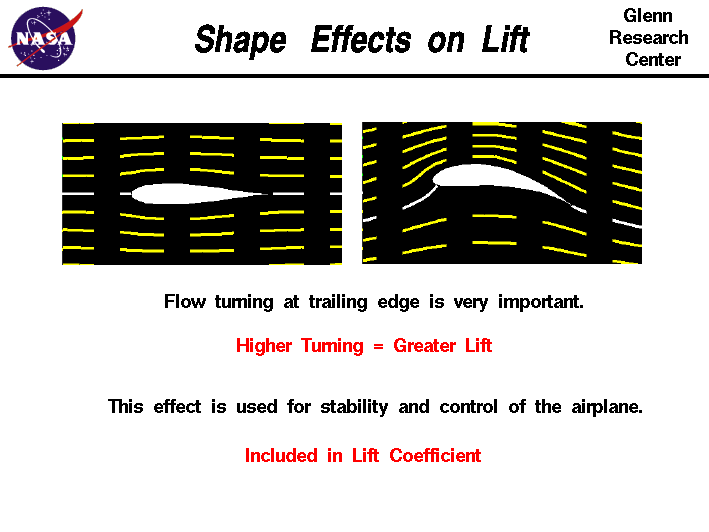
The approach fuses multifidelity data from disparate information sources available to designers, such as disciplinary simulations, experiments, and operational data from previously deployed systems. This paper presents a methodology to support improved decision making and identification of risks in early stage multidisciplinary design.
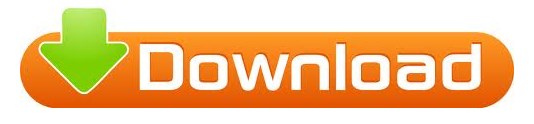